In this guest blog written by Harry Bowles and Sarah Opie-Martin from King’s College London they discuss their MND research poster which was presented at the European Network to Cure ALS (ENCALS) meeting in June 2022.
Randomised, double blind trials are the gold standard
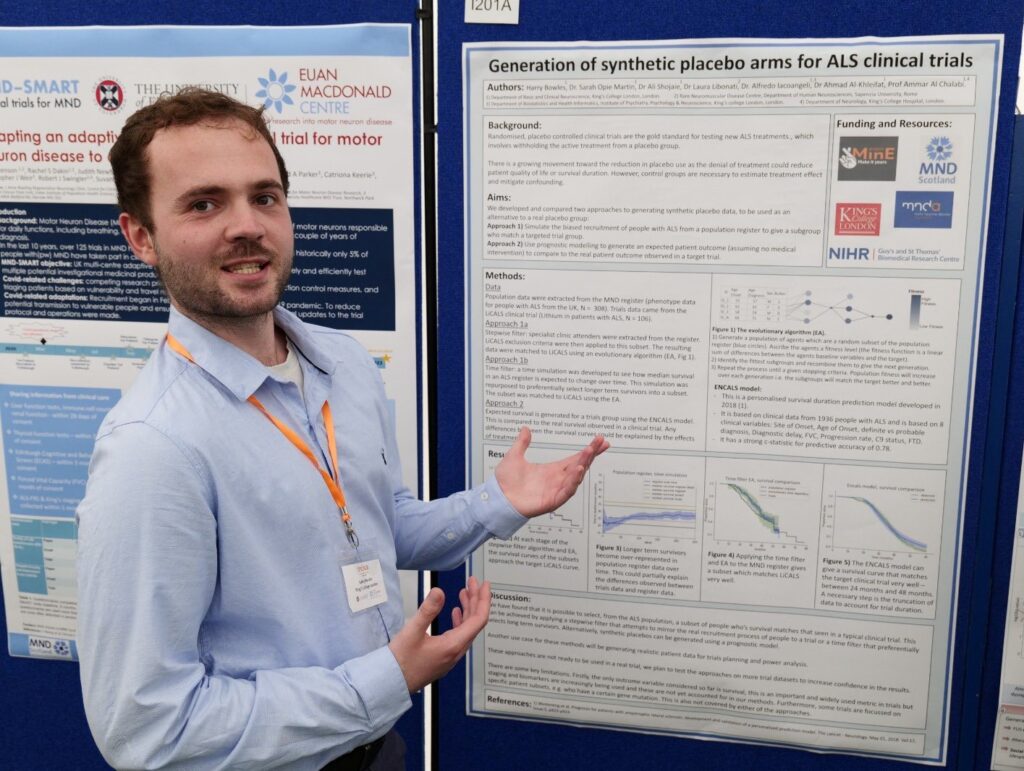
During a clinical trial, a group of volunteers with ALS are randomly divided into either a treatment or placebo group. The treatment group receives the real experimental treatment while the placebo group receives an inactive treatment. The placebo group acts as a control; if the disease progression of the real treatment group is significantly slower than the placebo group, the researchers can conclude that the experimental treatment is indeed effective. Neither the scientists running the trial or the participants themselves know who is in each group, this greatly reduces subconscious human biases.
Placebo groups are an essential part of clinical trials as they help to understand if the treatment is beneficial for people living with ALS. There are many discussions about the ethics surrounding the use of placebo groups within clinical trials. With some believing that withholding the experimental treatment from the placebo group is unethical. However, not having a placebo group can make it difficult to know if the treatment has been effective and could lead to a disruption in regulatory approval processes. These discussions have made an impact in recent clinical trial design. Many trials now offer an open label extension to placebo-controlled trials. These open label extensions happen after the placebo-controlled period of the trial. They give all participants on the trial the option to take the treatment regardless of whether they were on the treatment or placebo during the placebo-controlled period.
Novel methods to generate ‘synthetic placebo data’
In a clinical trial, the progression and survival duration of the experimental and placebo groups are recorded, and this data forms the basis of the statistical tests that help researchers to determine whether a treatment is effective or not for people living with ALS.
Understanding survival data graphs, such as those shown in figure 1, is pivotal in understanding outcomes from clinical trials. As time goes by, the proportion of participants who are still alive decreases, which is shown by the blue and green curves. We can test using statistics to see if there is a significant difference between the survival curves of the blue treatment group and the green placebo group. In figure 1a, there is a clear difference between the two groups with participants in the treatment group living longer than participants in the placebo group – this suggests that the treatment is effective. There is no difference in figure 1b, suggesting the treatment does not increase the life span of people with ALS. Figure 1c represented an open label extension trial. We can see the two curves begin to separate, but then, at 18 months, the control group are given the treatment and the curves begin to come together. This could suggest the treatment is effective, though it is harder to interpret.
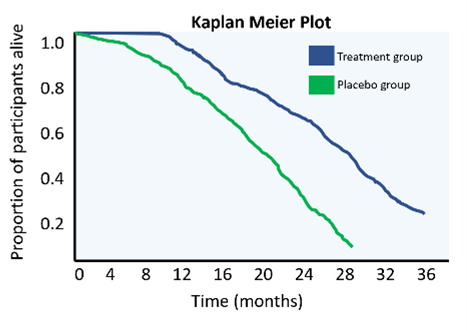
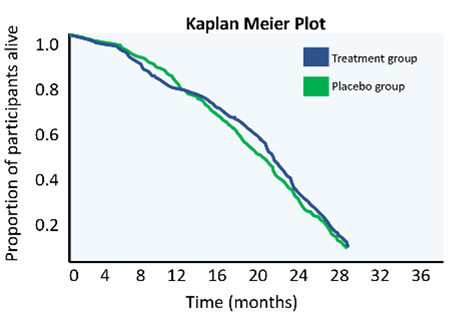
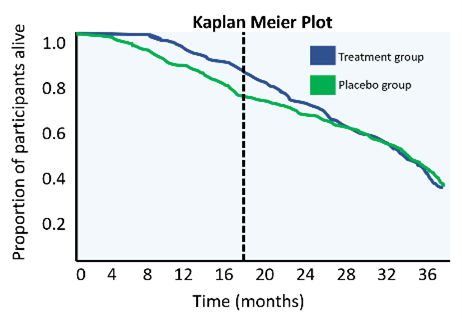
Figure 1. These ‘Kaplan Meier’ plots describe the survival duration of participants in an ALS trial
In this project we attempt to generate survival duration data using artificial intelligence (AI) and statistics, as an alternative to enrolling a placebo group. This data could be used during the planning stages of a trial to more accurately estimate sample sizes or ‘statistical power’ – which could improve trial efficiency. In the future, if the methods are proven robust and unbiased, it might also be possible to supplement real placebo groups with synthetic data to reduce the number of placebo participants needed in trials.
We are still in the very early stages of this research and none of the following information has yet been peer reviewed or published anywhere else.
Two different approaches to generate this synthetic data
The first approach uses population register data, which is known as the MND register, and applies a set of filters and algorithms to produce a data subset that matches a target clinical trial.
The population register contains clinical information about people with ALS in the UK. The population of people with ALS is heterogenous, meaning the clinical symptoms and underlying genetics of each person with the disease can vary. There are also systematic biases that cause the participants in clinical trials to be statistically different to the general ALS population. E.g. trial participants tend to be younger and more males than females. This means we simply can’t compare the population register data with trial data. To account for this heterogeneity and the recruitment biases, we have developed the ‘evolutionary time’ algorithm to select biased subgroups from the population register to target a given clinical trial. This allows us to match the two groups based on clinical variables such as mean age of onset and sex.
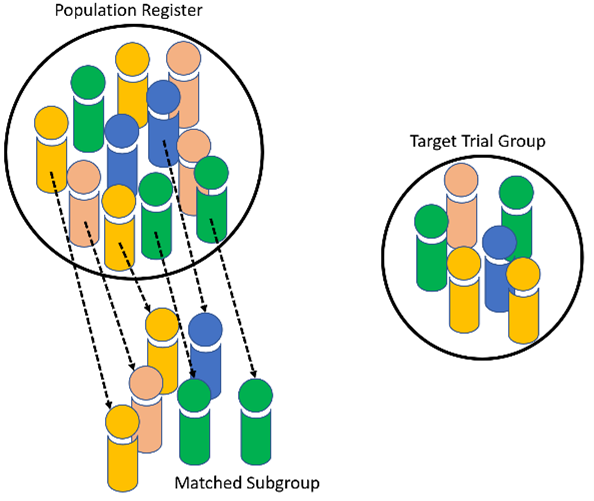
This custom ‘evolutionary time’ algorithm (figure 2) was used to select from the register a biased subset whose clinical characteristics matched those of a previously run clinical trial.. The matched subset is the ‘synthetic placebo’ group. We can then compare the survival curves of the synthetic placebo group to see if it matches the survival duration curve of the real placebo group. If it does match, then we will know that this method is capable of producing realistic survival data that can be targeted to a given trial. We found that the survival curves of the synthetic data do indeed match the placebo data very well.
In the second method we used a model that predicts survival of people with ALS based on information collected at their first appointment such as the age their symptoms started and where their symptoms started. We compared the predicted survival of people in the placebo group with the real survival data that was recorded by trial investigators. We found that the survival is very similar between the groups when modelled in the first two years after trial enrolment.
Both methods were found to provide synthetic placebo groups that matched placebo groups from a completed trial. There is potential for these synthetic placebo groups to be used to supplement and reduce the number of participants randomised to placebo arms. This would allow more people with MND to be given the treatment in clinical trials and perhaps increase participation in trials since the chance of being in the placebo arm would be greatly reduced. However, this work is still in the very early stages and needs to be tested on many more datasets before being applied in real world trials.
We wish to thank Harry and Sarah for writing this blog.
Further reading:
Evolutionary algorithms: https://towardsdatascience.com/introduction-to-evolutionary-algorithms-a8594b484ac
ENCALS model: https://bmcneurol.biomedcentral.com/articles/10.1186/s12883-020-02004-8