In June, we hosted the second MND EnCouRage UK event for early career researchers (ECRs) which aims to support them to continue working in the field of MND. The event included lots of talks and workshops from senior researchers to provide tips and advice on moving forward in their careers and help them to develop new skills in areas like presenting their work to non-scientific audiences. We also challenged the ECRs to write a blog to explain their research to the MND community. This guest blog is from Florence Townend, one of the ECRs who came to the event this year.
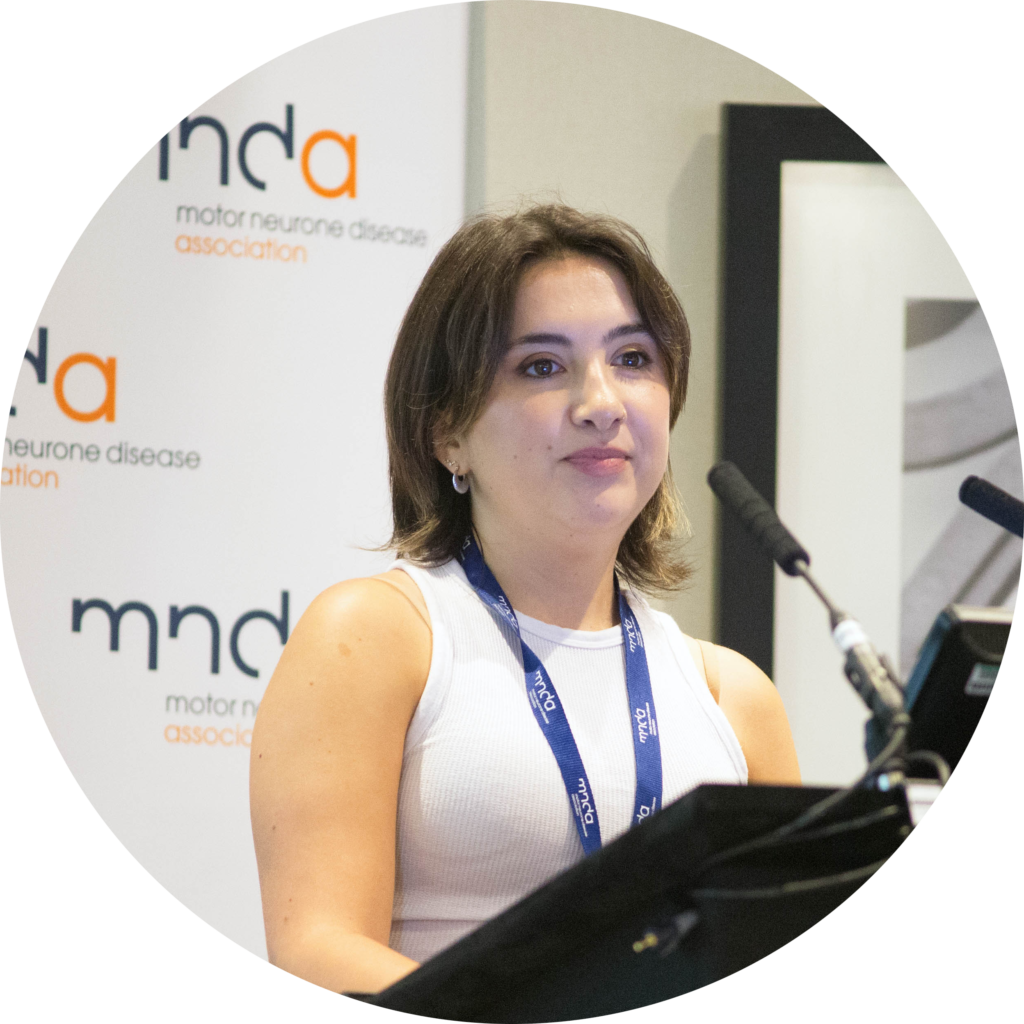
My name is Florence and I’m doing a PhD in artificial intelligence (AI) enabled healthcare at University College London. My research is specifically investigating how AI techniques can be used to improve the prediction of how quickly somebody’s disease will progress in motor neurone disease.
I’m originally from Reading in Berkshire and, for my undergraduate degree, I studied Engineering Mathematics at the University of Bristol. During my time there, I mainly specialised in the fundamentals of AI and how we can simulate human decision-making with AI models of the brain.
After reading and studying a lot about the brain for my undergraduate research project, I became interested in how AI can be applied to other aspects of neuroscience. I decided to enrol in a Master of Research and a PhD in AI-enabled Healthcare at University College London, where I am investigating the application of AI in the context of neurodegenerative conditions, specifically MND.
My PhD Research
A lot of data is generated in clinical care, for example GP notes, MRI scans, blood test results, prescription records and , patient histories.. Let’s say that I have all this data available to me about somebody and I want to predict whether they will develop a disease in the next 5 years. Even with medical expertise, trawling through the various data sources and picking out parts I think are useful, and then trying to combine the information from the clues I’ve found to finally come up with a single answer would be time-consuming and challenging.
I could make my life a lot easier if I just look at their blood tests and make my prediction based on that. However, I would miss out on all the other information I have completely ignored, which might mean my prediction isn’t very good.
So, how can we combine lots of different types of data for accurate predictions, without it being completely impractical? My PhD research aims to solve this problem with AI, specifically in the context of predicting prognosis of motor neurone disease.
Traditionally in motor neurone disease, how quickly somebody’s disease will progress is estimated by how quickly their ALSFRS-R score declines. There has been research into other tools for predicting prognosis such as the ENCALS model, which includes a more comprehensive set of clinical features, and there has also been interesting work into how genetic risk factors, brain scans, and spinal cord scans can provide insight on how somebody’s future looks with MND. Additionally, quite a few different data types are recorded leading up to an MND diagnosis.
Therefore, I am researching how these different data types often available at MND diagnosis (clinical features, brain scans, blood tests) can be combined using AI in order to predict disease progression.
What does it mean for people living with and affected by MND?
Accurately predicting the prognosis of MND is of utmost importance for optimising clinical trial design and effectively managing people’s care. People living with and affected by MND often voice their frustration over the uncertainties surrounding disease progression, which presents significant challenges in planning for the future.
The end goal for my research is to create a computer tool that a clinician or healthcare practitioner can use to get a prediction of how quickly a newly diagnosed person’s MND will progress.
I do acknowledge that getting the tool into actual clinical use during my PhD may not be feasible due to the important rigorous safety checks, regulations, and logistical complexities involved. However, I believe that by laying the groundwork and exploring the potential of AI in predicting MND prognosis, we’re moving closer to a future where a deployable tool may become a reality.
Secondary outcomes of my work could be found through more in-depth analysis of experiment results, such as understanding which types of data are the most useful for accurately predicting prognosis, and if the interactions between the information in different data types can shed some light into what drives slow or fast progression.
With more clinical trials looking at possible treatments of MND, there is a larger focus on finding ways to measure and predict disease progression more accurately. Clinical trials could be better designed so that treatments could be tested in a specific group of people with MND, with a specific rate of progression, who the treatment may be most effective for. We hope this work will help to increase our understanding of disease progression so that this may be more accurately predicted for people with MND and help to improve the design of clinical trials so that potential treatments can be given the best possible chance of success.
We would like to thank Florence for taking the time to write this guest blog about her work and also thank everyone involved in making MND EnCouRage UK 2023 such a success.